Adaptive Robotic Carving
In recent decades, digital fabrication technologies have become increasingly available, yet manufacturing knowledge is rarely integrated within the established workflows of design practices. Materialisation processes are regarded as the last stage of design-to-manufacturing workflows, where materials are considered passive receivers of a previously-generated ideal form, stored in a digital model. Such a linear progression from the design intention to its materialisation necessarily limits the feedback between different stages of the process, forcing designers to engage with only a limited range of standard manufacturing methods and materials, which often leads to wasteful and inefficient solutions. The complex interaction of non-standard tools, such as chisels and carving gouges, with the heterogeneous properties of timber, cannot be ignored within robotic manufacturing, as it substantially affects the resulting carved geometry.
The central challenge addressed in this research was to develop an adaptive simulation model that could bridge the digitally-prescribed design intention and the outcome of the carving process. The research proposed a series of methods to capture, transfer, augment and integrate manufacturing knowledge at an early stage of the design process through the collection of real-world fabrication data, using different sensor devices and machine learning models to achieve an accurate prediction of carving geometries, informed by material behaviours.
The acquisition of fabrication data was structured by a series of recording sessions to store, in a library of datasets, the combination of fabrication parameters and respective operation outcomes generated by different material properties (e.g. grain structure, density, direction), wood species and carving tools. The collected datasets can be used to train multiple Artificial Neural Networks (ANNs), whose main learning objective is to predict the carved geometry generated by a user-defined robotic toolpath and a series of fabrication parameters.

Collaborations with two industry partners – ROK Architects, Zurich, and BIG, Copenhagen – provided the opportunity to apply the methods we had devised into established workflows and develop a catalogue of design explorations for a wide range of applications, from furniture to building components of larger assemblies. The curation of the training process, by a team of designers, represents the keystone of the design workflow, as the selection of relevant material affordances and fabrication parameters directly determines the range of solutions later available in the digital design exploration. In this way, the trained system represents a package of knowledge that can be integrated within an interface to digitally evaluate multiple, otherwise unavailable, design solutions informed by tools and material properties, before moving to the production stage. Once robotically fabricated, the selected carved geometries are compared in a deviation analysis with the respective simulations, to assess the predictive abilities of the system.
The results successfully demonstrate the ability of the trained networks to accurately model the outcome of carving operations, defined in the digital design environment by a series of fabrication parameters. The impact of the research lies in devising a series of robotic training methods that allow for the flexible extension of the range of subtractive manufacturing processes available to designers.
Novel design opportunities are explored to support decision-making procedures, based on an accurate simulation of non-standard operations on timber. The integration of manufacturing knowledge at the early design stage allows for a custom design-to-manufacture workflow to be established, informed by continual feedback, that encourages a fruitful dialogue between designers and manufacturers.


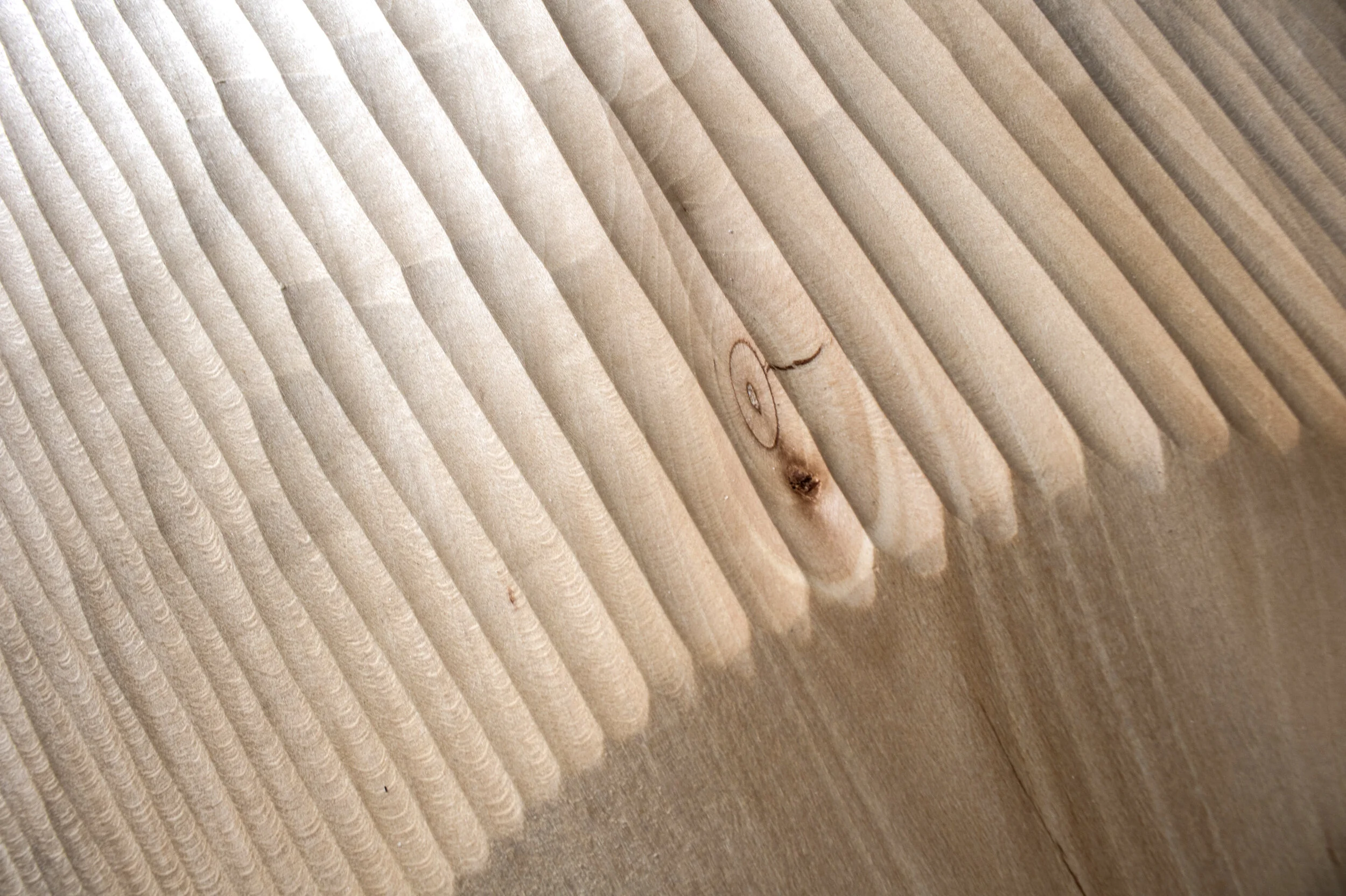

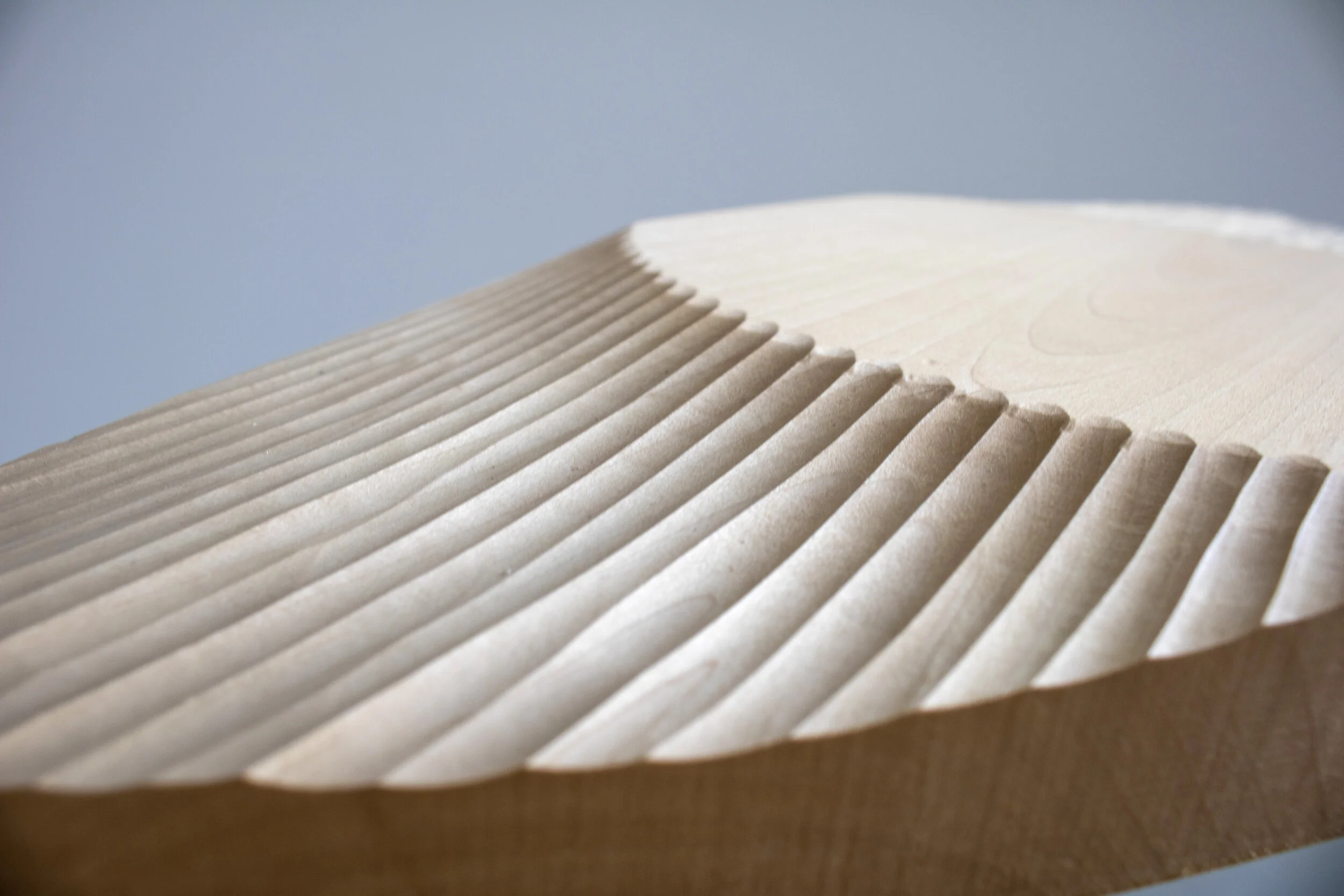
